 |
|
|
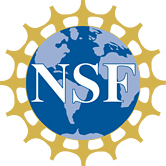
|
NSF Project on Quantum Machine Learning Tools
PI: A. Spanias and Co-PI: W. Barnard, Quantum Machine Learning Tools, NSF 2215998, Sept. 2022-Sept 2025.
|
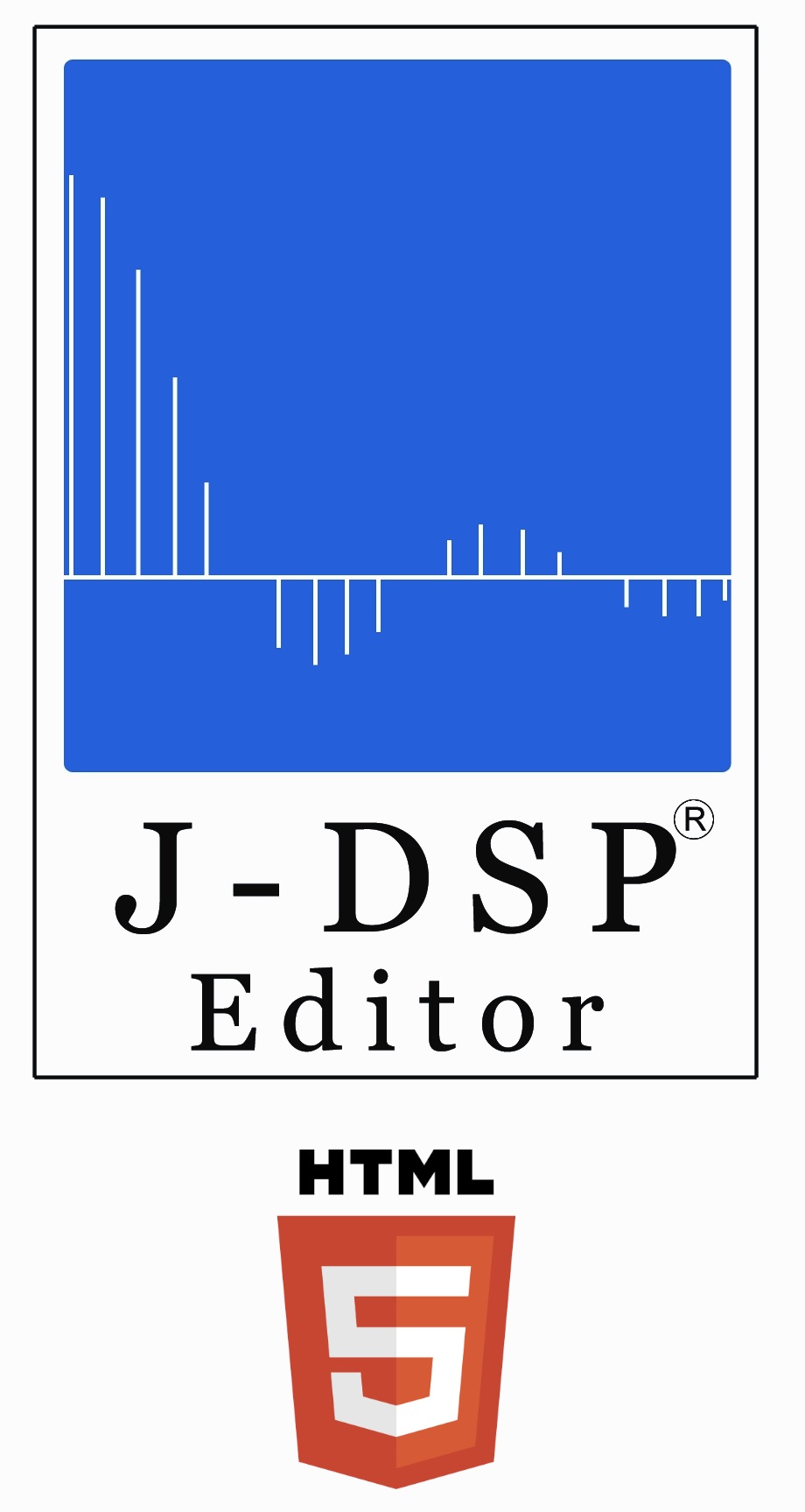
|
In this IUSE Level 1 project, we focus primarily on creating user-friendly quantum machine
learning tools for research and education. Tools will be deployed in classes, workforce
development programs, capstone projects and research projects. These tools will enable researchers
and students with diverse backgrounds and skill levels to explore the potential applications
of quantum machine learning, without requiring them to have extensive knowledge of quantum mechanics or programming.
From these quantum functions, the students can obtain experiences
on quantum precision, quantum noise, and quantum signal
processing in a relatively short time without having to learn
an entirely new programming environment.
|
We expand the J-DSP functionality by developing user-friendly Quantum Computing simulation functions for Fourier transforms. These functions are intended to provide hands-on laboratory experiences within the DSP class. We focus on signal analysis-synthesis using the Quantum Fourier Transform where students simulate a simple compression scheme
using quantum computing. The QFT based signal analysis synthesis simulation video is shown below. Further details are mentioned in this link.
During the QFT based signal compression exercise given to the undergraduate students, we conducted a pre and post quiz evaluation to assess the impact and effectiveness of the exercise. The evaluation results are shown in the graph figure below.
To analyze the results, we compared the number of correct answers between the pre and post quizzes. This allowed us to evaluate the level of knowledge improvement achieved by the students after participating in the quantum computing exercise.
The graph illustrates the effectiveness of the exercise in enhancing students' understanding of the quantum computing. We observed a noticeable increase in the number of correct answers in the post-quiz compared to the pre-quiz indicating the
significant improvement in the students' knowledge and comprehension of the quantum computing, signal analysis synthesis and quantum Fourier transform concepts following their participation in the exercise. This evaluation shows that students
gained knowledge on introductory QC concepts and QFT
complexity (Q.2. and Q.3.), quantum noise effects (Q.1.), the
effect of the number of qubits (Q.4.), and the effect of
selecting QFT components (Q.5.) in signal analysis synthesis. The average improvement observed in the postquiz is around 46%.
|
Ongoing research in quantum computing :
L. Miller, G. Uehara, A. Sharma, A. Spanias, "Quantum Machine Learning for Optical and SAR Classification,"
IEEE DSP Conference, Rhodes, June 2023
A. Sharma, G. Uehara, V. Narayanaswamy, L. Miller and A. Spanias, "Signal Analysis-Synthesis Using The
Quantum Fourier Transform," 2023 IEEE International Conference on Acoustics,
Speech and Signal Processing (ICASSP), Rhodes Island, Greece, June 2023.
G. Uehara, V. Narayanaswamy, C. Tepedelenlioglu, A. Spanias, "Quantum Machine Learning for
Photovoltaic Topology Optimization," 2022 IEEE 13th International Conference on Information,
Intelligence, Systems & Applications (IISA), Hybrid Conference, July 2022.
M. Yarter, G. Uehara, A. Spanias, "Implementation and Analysis of Quantum Homomorphic
Encryption," 2022 IEEE 13th International Conference on Information, Intelligence, Systems &
Applications (IISA), Hybrid Conference, July 2022.
Glen Uehara, Jean Larson, Wendy Barnard, Michael Esposito, Filippo Posta, Maxwell Yarter, Aradhita
Sharma, Niki Kyriacou, Matthew Dobson, Andreas Spanias, "Undergraduate Research and Education
in Quantum Machine Learning," IEEE FIE 2022, Upsala, Oct. 2022.
G. Uehara, A. Spanias, W. Clark, "Quantum Information Processing Algorithms with Emphasis on
Machine Learning," Proc. IEEE IISA 2021, July 2021.
|
|
|